Obsess Over Controllable Input Metrics
Why Amazon's notion of measuring controllable input metrics is a lot more profound than you might think.
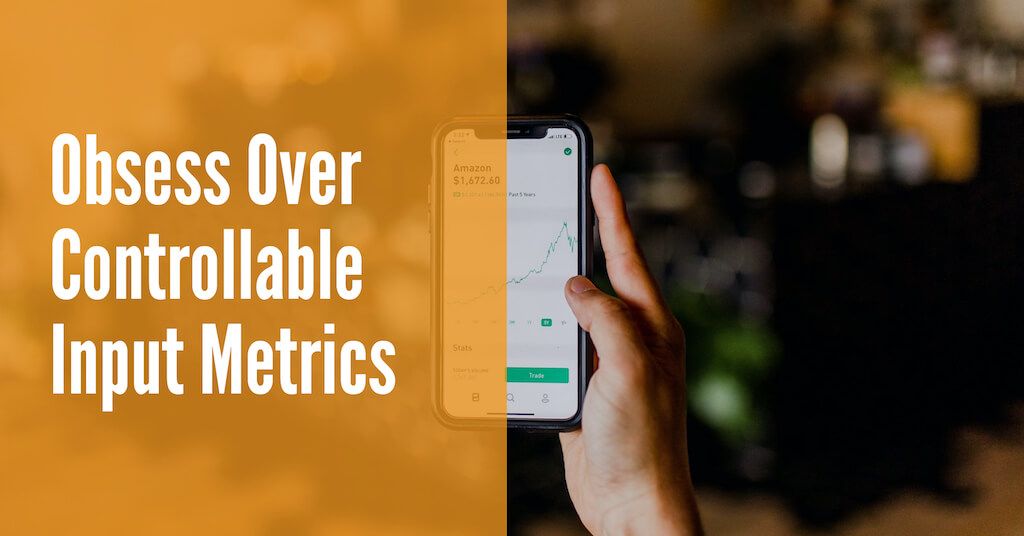
I’ll admit it: I’m a little obsessed with Amazon’s concept of controllable input metrics.
The idea itself seems trivially simple. “So?” I can hear you say. “Isn’t controllable input metric just a fancy way of saying ‘leading indicator’? And isn’t it just another thing that you should already be doing when you set your OKRs?”
These are good points. But they are largely orthogonal to the style of thinking that controllable input metrics forces you to do. I would argue that controllable input metrics is the sort of idea that seems trivial on the surface, but then changes the way you think about data once you put it to practice. And in fact, I would go so far to say that it has fundamentally changed the way I think about operational rigor.
I want to give you a taste of this in this blog post. So let's begin.
Try It Out
The key to grokking the nuances of this idea is to attempt to come up with controllable input metrics for your own company.
Humor me. Think of some business process that you’re involved with. Now think about the metrics you might measure when instrumenting that process.
If you’re anything like me, you would think of metrics like these:
- Sales: number of deals closed.
- Marketing: blog traffic, or number of new newsletter subscribers per week.
- Software Engineering: number of new features shipped per time period.
- Customer Support: post-customer support survey scores / NPS.
And so on.
The odds are good that the metrics you pick are going to be output metrics — that is, outcomes that you want for the business, but that you actually have indirect control over.
Take blog traffic, for instance. Say your goal is to increase blog traffic by 2x within the next six months. What would you do? Write more guest posts? Do more SEO? Spruce up your site structure? Try and have several posts go viral?
The likely answer is that you’ll try all of these things, but with the understanding that you’ll experience a delay from your efforts. For SEO-related improvements, for instance, these may well be a six month gap between action and results.
And so measuring output metrics isn’t good enough. It’s not good enough if you want to motivate your people, and it’s certainly not good enough if you work at Amazon. As Colin Bryar puts it, if you want to be a good operator, you’ll need to have a concrete understanding of the factors that you do have control over, that contribute directly to the output metric that you care about. And you need to measure that. He argues:
"The best operators I've seen very clearly understand that if they push these buttons or turn these levers in the right way, they're going to get the results they want. They understand that process through and through.”
These input metrics are usually customer-related. "Is the customer experience getting better this week than it was last week? That's harder to figure out than it sounds. So you monitor 10 or 20 different things, you experiment a little bit," says Bryar. "Measure them day in and day out — a great operator always instruments, so they know exactly what's happening. If you don't measure something, it's going to go wrong."
What you’ll want to measure are controllable input metrics — things that you know that you can improve today, that would lead to changes in your desired output metric months down the line. These input metrics tend to incentivize behaviour if you set them up as organizational goals. So, for instance:
- For sales, you measure the number of responses/interactions from unique individuals that fit your ideal persona. (The targeted output metric here: number of deals closed).
- For marketing: % of blog posts with a custom incentive email form embedded within the text. (The targeted output metric here: number of new email subscribers).
- For software engineering: mean time from commit to deployment. (The targeted output metric here: number of new features shipped per time period).
- For customer support: % of tickets that are closed within 3 days. (The targeted output metric here: NPS).
The key property of many of these metrics is that you’ll stop and go “wait a minute, I don’t think these metrics would lead to the outcomes we want / I don’t think these metrics would work for us.” And that is the entire point. A controllable input metric is, by definition, a few layers before your desired output metric, and you would need to investigate if a firm relationship exists between the two. More importantly, a controllable input metric should lead to immediate behavioural change within your team, and you should feel fear that it would lead to some sort of misaligned incentives. (Think: a dysfunctional Goodhart’s Law-type situation).
Amazon argues that this is totally expected, which is why you have to go through a period of trial and error, in order to see if the input metric leads to the outcomes you desire. A huge part of my summary of Working Backwards talked about Amazon’s process for picking controllable input metrics. They called it ‘going through the metrics lifecycle’, and they named their metrics selection process DMAIC, or ‘Define, Measure, Analyze, Improve and Control’.
So: I hope you’re still with me. I hope you’ve tried picking a plausible input metric for your business process, along with a commensurate output metric it is supposed to influence. To provoke you a bit more: try going through a number of different processes in your business. For each business process, ask:
- What’s the desired behaviour that we want here? (e.g., for the data team, this might be — we want to increase the % of business decisions that are made with data).
- What’s our output metric for that, and how do we instrument it? (How are you going to capture if decisions are made with data or not?)
- What’s our input metric? A possible candidate is: % of internal reports that are accessed — be it via dashboard or read email — at least once a week.
- Now prepare to go through the DMAIC trial-and-error process that Amazon uses, to check that your chosen input metric really does affect the output metric.
Rinse and repeat for the rest of the processes you want to workshop in your head.
Why Is This Powerful?
So let’s circle back to my original question: why is this important? And why do I find the idea so profound?
The simple answer is that we are not taught to think like this. When people say “be more data driven”, we immediately assume, “oh, we have to measure our business outcomes”. And so we measure things like number of deals closed, or cohort retention rates, or revenue growth, or number of blog visitors. These are all output metrics — and while they are important, they are also not particularly actionable.
Amazon argues that it’s not enough to know your output metrics. In fact, they go even further, and say that you shouldn’t pay much attention to your output metrics; you should pay attention to the controllable input metrics that you know will affect those output metrics. It is a very different thing when, say, your customer support team knows that their performance bonuses are tied to a combination of NPS and ‘% of support tickets closed within 3 days’. If you have clearly demonstrated a link between the former and the latter, then everyone on that team would be incentivised to come up with process improvements to bring that % up!
Of course, there are potential problems with this approach. But Amazon has developed a number of techniques to prevent misaligned incentives:
- Every metric has an owner, and every metrics owner is expected to understand what is normal variation and what is an anomaly.
- Metrics are audited by an independent department (finance), which prevents business owners from juicing metrics, or picking metrics that make them look good.
- Metrics are reviewed every week, at the Weekly Business Review meeting. (This occurs fractally — at the top level all the way down to each team).
- The finance department informs both leadership and business owners how they’re tracking for each of their yearly goals, on both input and output metrics.
- Execs and business owners are expected to critically evaluate the effectiveness of every metric, and are allowed to modify or remove them if they are no longer useful.
- Metric owners must have a process for auditing each metric, to check that it measures what it’s supposed to be measuring. The audit process triggers on a periodic basis.
The point I’m trying to make is that Amazon uses metrics as a finely tuned operational weapon — and that it understands, from an incentives design perspective, the power well-designed metrics can have over organizational behavior.
In the Business Intelligence world, we often spend time talking about cool new tools, or new pipelining tricks, or data unit tests, or ‘notebooks, not dashboards!’ But arguably, none of that matters if your org isn’t set up for data-driven decision making.
Amazon ran its original metrics meetings with decks of printed paper. And they did this in the early 2000s, when columnar data stores were nascent, and OLAP cubes were still the norm. And it succeeded beyond its wildest dreams.
So: what do we have to say for ourselves?
What's happening in the BI world?
Join 30k+ people to get insights from BI practitioners around the globe. In your inbox. Every week. Learn more
No spam, ever. We respect your email privacy. Unsubscribe anytime.