The Four Flavors of Goodhart's Law
Goodhart's law says that 'when a measure becomes a target, it fails to become a good measure. Here are four ways that occurs.
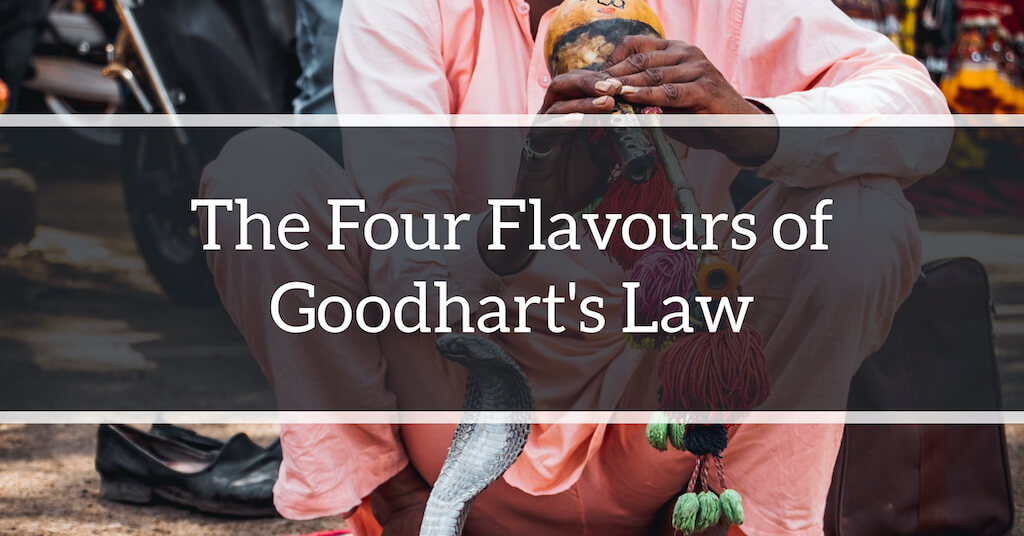
Goodhart’s law is a famous saying named after the British economist Charles Goodhart, which usually goes “when a measure becomes a target, it ceases to be a good measure.”
This idea is of interest to businesspeople, managers, and data analysts alike — and for good reason: companies are usually run using metrics, and few things are worse than a well-meaning metric turned bad.
A famous example of this is what is now called the ‘cobra effect’. The story goes as follows: in India, under British rule, the Colonial government was concerned about the number of venomous cobras in Delhi. The government thought it was a good idea to recruit the local populace in its efforts to reduce the number of snakes, and started offering a bounty for every dead cobra brought to its door.
Initially, this was a successful strategy: people came in with large numbers of slaughtered snakes. But as time passed, enterprising individuals began to breed cobras with the intention of killing them later, for the extra income.
When the British government discovered this, they scrapped the bounty, the cobra breeders released their cobras into the wild, and Delhi experienced a boom in hooded snakes.
The Raj’s cobra problem was hence no better than when it began.
The Four Forms of Goodhart’s Law
Today, we know that there are four flavors of Goodhart’s law. David Manheim and Scott Garrabrant laid out these flavors in their paper, Categorizing Variants of Goodhart’s Law; Manheim later wrote another paper titled Building Less Flawed Metrics, which he distributed through the Munich Personal RePEc Archive. (I should note that none of these papers are peer-reviewed, but I do not think that detracts from their value.)
In their paper, Manheim and Garrabrant lay out their case for the four categories in an extremely generalized manner — which makes sense, once you think about the applications of Goodhart’s law. For instance, Manheim and Garrabrant were interested in the implications of the idea on AI research (imagine if you told a super intelligent AI to optimize paperclip manufacturing, and it decided to liquify humans in order to produce more paperclips …), but a better understanding of Goodhart’s law is broadly applicable to public policy, company management, and incentive system design.
This post is a layperson’s summary of the four flavors; it is not meant to be an exhaustive accounting of all the sub-cases in the paper. But a broad understanding of the four buckets should prove useful to the average businessperson, I think. Let’s get started.
Regressive Goodhart
The first flavor of Goodhart’s law is the only one that is impossible to prevent.
Let’s imagine that you have to hire candidates for a job. What you really want to measure is their future job performance — but you can’t measure this directly during the job interview. Then, you learn that IQ is correlated to job performance at about 0.6. You decide to administer an IQ test instead. What could go wrong?
At first, the test is a success — your company manages to hire people who are better than those who were hired through the old process. Encouraged by this, you slowly begin to optimize your hiring for IQ and IQ alone: for instance, you advertise that your company is incredibly selective, wonderful to work for, filled with smart people, and so on. But after some time, you realise that the people with the highest IQs tend to perform worse than some of the above-average candidates. And some of these high-IQ people are downright unpleasant to work with! Congratulations: you’ve just experienced regressive Goodhart.
Regressive Goodhart occurs because the measurement you’re using as a proxy for your goal is imperfectly correlated with that goal. In our example above, IQ has a correlation of 0.6 with job performance, which is a good correlation by social science standards, but then it also means that there are other factors that matter for job performance. By optimizing for IQ alone, you’re likely to get suboptimal outcomes, because you ignore those other factors.
To understand why this might be true, let’s say that the psychological trait of conscientiousness is also a predictor of future job performance (it is, just in case you’re wondering). If you’ve optimized for people with super high IQs, you are essentially picking from a small pool of people, since there are fewer people with super high IQs in any population. The odds that you will pick someone from that small pool who also has high conscientiousness is really low; therefore we should see a result where people with the best job performances have higher-than-average IQs, but people with the highest IQs do not have the best job performances (since they are unlikely to also have high conscientiousness, and conscientiousness contributes to job performance). This effect is sometimes known as ‘the tails comes apart’.
In practice, regressive Goodhart is impossible to avoid because nearly every measurement you can think of is an imperfect reflection of the true thing you want to measure. If that metric becomes a target, then you are likely to drift from your true goals.
What do you do about this? One solution might be to pair opposing indicators, as legendary Intel CEO Andy Grove once suggested. But another way, one that Manheim suggests, is to look for more accurate measurements of your true goal — easy to say, but hard to do!
Extremal Goodhart
Extremal Goodhart occurs when you pick a measurement because it is correlated to your goal in normal situations. But then adopting this measure then makes you optimize for that measure, and at the extreme ends of that measure, the relationship with your goal breaks down.
Garrabrant gives an example of our relationship with sugars: humans evolve to like sugars, because sugars were correlated with calories in our ancestral environment. This worked great when we were hunting lions; today, however, this same optimization leads us to drink Coke and eat Doritos and slide into obesity.
In machine learning, this sometimes happens due to ‘underfitting’. For example, a relationship between two variables is assumed to be a low degree polynomial because higher order polynomial terms are small in the observed space. Then, selection on the basis of this metric moves towards regions where the higher-order terms are more important, so using the machine learning system creates a Goodhart effect.
Causal Goodhart
You are a principal of a high school. You learn that students with good high school exam scores do better on college exams. You conclude that helping your kids do well on their high school exams will lead to good things, so you roll out a program to teach them test-taking skills. You also pressure your class teachers to funnel students to easier subjects, because that increases their average exam scores.
It doesn’t work. You’ve just experienced causal Goodhart.
Another, more trivial example: you are a kid. You read that basketball players are more likely to be tall. You want to be tall. Therefore you play basketball.
This particular flavor of Goodhart’s law is easy to understand. The idea is that you think that a measure produces an outcome when actually the two are correlated (and may be caused by some third factor). Naturally, if you optimize one or the other, you usually do not affect the outcome you want. In our exam example above, it’s clear that high school exams predict college exams only in so far as they are a reflection of the intelligence, knowledge, and hard work of the students (amongst other things). Trying to achieve better college results by juicing high-school test taking ability is of limited usefulness at best.
This idea is what people mean when they say “correlation does not imply causation.”
Adversarial Goodhart
Adversarial Goodhart is the story of the cobras under the British Raj, above.
Wikipedia’s article on the Cobra effect has a number of other entertaining examples, including this one:
In 1902, the French colonial government in Hanoi created a bounty program that paid a reward for each rat killed. To collect the bounty, people would need to provide the severed tail of a rat.
Colonial officials, however, began noticing rats in Hanoi with no tails. The Vietnamese rat catchers would capture rats, sever their tails, and then release them back into the sewers so that they could procreate and produce more rats, thereby increasing the rat catchers' revenue.
A related example of an adversarial Goodhart is Campbell’s law:
"The more any quantitative social indicator is used for social decision-making, the more subject it will be to corruption pressures and the more apt it will be to distort and corrupt the social processes it is intended to monitor."
You can imagine a situation where a government says that all of its policies must be based on ‘evidence’, and so therefore this results in great pressure (and plenty of incentive!) for the various players in the system to manipulate, polish, and massage ‘evidence’ in order to justify government policies.
The general idea here is that an agent may optimize for a metric in a way that defeats the metric’s goal (the Cobra effect), or the agent may choose to optimize for a measure in a way that reduces that measure’s predictive effect.
The solution? Manheim suggests engaging in ‘pre-mortems’, as in, “ok, this policy we’re about to choose has gone wrong in the future, what happened?” He points out that in a group, there doesn’t need to be that many people before someone comes up with a plausibly horrible scenario.
So there you have it: four flavors of Goodhart’s law. If there’s nothing else you remember from this essay, then remember this: if you want less snakes in your backyard, don’t pay for dead snakes.
What's happening in the BI world?
Join 30k+ people to get insights from BI practitioners around the globe. In your inbox. Every week. Learn more
No spam, ever. We respect your email privacy. Unsubscribe anytime.