Beware What You Measure: The Principle of Pairing Indicators
When you're implementing company-wide analytics, it's easy to fall into the trap of measuring only one metric. Don't. The principle of pairing indicators is why.
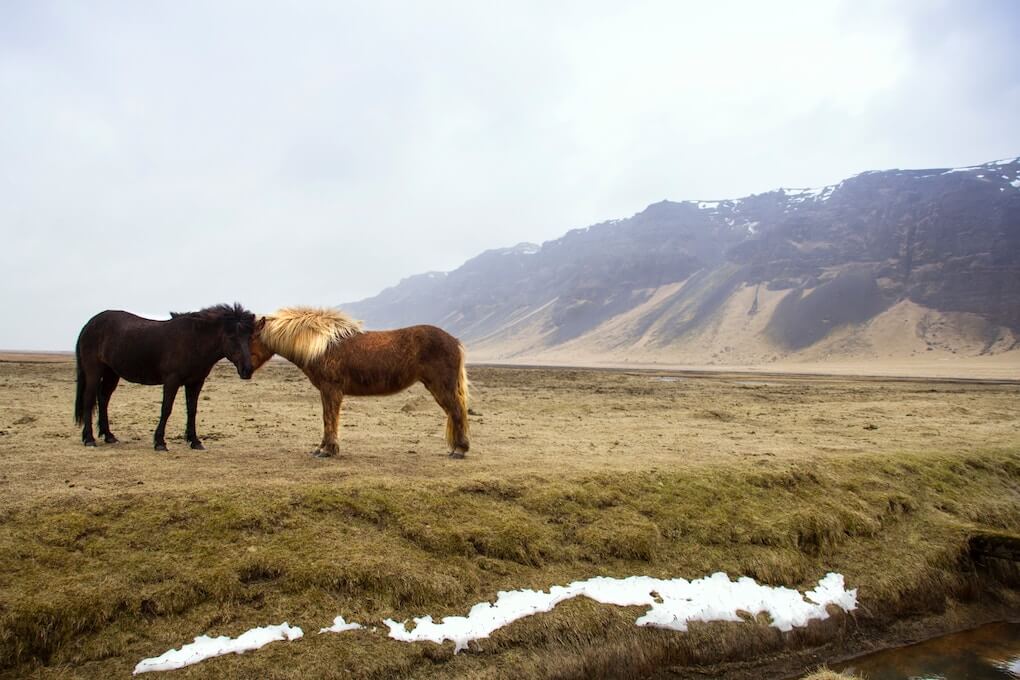
Let’s say that you want to measure the performance of your team. From an operations perspective, there are a ton of things you could measure that would give you a leading indicator of your team’s performance:
- If you’re in sales, you could measure number of deals closed per week.
- If you’re in engineering, you could measure number of assigned tasks completed per week. (Or some function thereof — you probably want a metric that combines difficulty of task with number of completed tasks … hence the concept of ‘story points’ in agile project management.)
- If you’re in customer support, you could track the number of support tickets handled per week.
Picking a single metric like this is the easy thing to do. It’s also a terrible, terrible idea.
You Make What You Measure
Over the years, one aspect of organisational behaviour that I've always found odd is the idea that organisations will optimise what it measures — regardless of the consequences!
The idea that you naturally optimise the things that you measure is often used in positive ways: for instance, startup investor Paul Graham argues that ‘you make what you measure … so pick your measurement carefully’. The ride-hailing company Uber measures nearly everything they do by its impact on their Gross Merchandising Value — giving the entire company a single number around which to build all their growth and product initiatives.
But there is also a downside to this notion of ‘making what you measure’. Consider our list of metrics above. How would you feel if your company optimised those metrics to the extreme?
- Your sales team optimises the number of deals closed … to the extent that they sacrifice retention. They close bad-fit customers who don’t get value out of your product. A large percentage of these customers churn a few months later.
- Your software engineering team optimises the number of difficult tasks delivered … to the extent that they sacrifice code quality. This results in technical debt that hampers your product’s development speed in the future.
- Your customer support team optimises the number of support tickets handled per week … at the expense of customer happiness.
Why does this happen? The reason this happens is because it is terribly easy for teams to forget the purpose of a metric, and instead organise activities around the optimisation of the metric itself. Over time, the original purpose of the measurement gets lost. This is especially true if the metric is tied to performance bonuses.
Amazon CEO Jeff Bezos calls this ‘making the process the thing’. Legendary Intel CEO Andy Grove calls this ‘… like riding a bicycle: you will probably steer it where you are looking.’
Good companies fight against this; you should too.
The Principle of Pairing Indicators
How do you solve this problem? The solution to this problem is to pair indicators — meaning you combine the measurement of an effect with a measurement of its counter-effect. This idea was originally presented in Andy Grove's seminal book High Output Management. So:
- If you measure number of deals closed, this metric should be presented alongside retention.
- If you measure number of difficult engineering tasks performed, that should be paired with the rate of new bugs per release.
- If you measure number of support tickets handled, you should pair that with your NPS score.
There are also a number of combinations of effect and counter-effect:
- You could pair a short-term benefit against a long-term cost.
- You could pair a quantitative measurement with a qualitative one.
- You could pair a process metric (number of sales calls made) against an outcome-driven one (number of deals closed).
Once you understand that metrics can be easily warped by institutional behaviour, you’ll be a lot more wary of single-metric measurements. Surprisingly, a huge violator of this principle are governments, who often choose single-metric measurements as the method with which to evaluate policy success.
The next time you observe an organisation measuring success according to a single metric, ask yourself: what’s the counter-effect here? What’s likely to go wrong if this metric is optimised to the nth degree? More often than not, I think you’ll be surprised at the answer. And you’ll be well-equipped to implement effective analytics in your own company.
What's happening in the BI world?
Join 30k+ people to get insights from BI practitioners around the globe. In your inbox. Every week. Learn more
No spam, ever. We respect your email privacy. Unsubscribe anytime.