Five Things We're Pretty Sure About
Five quick things we know to be true, written for the end of the year.
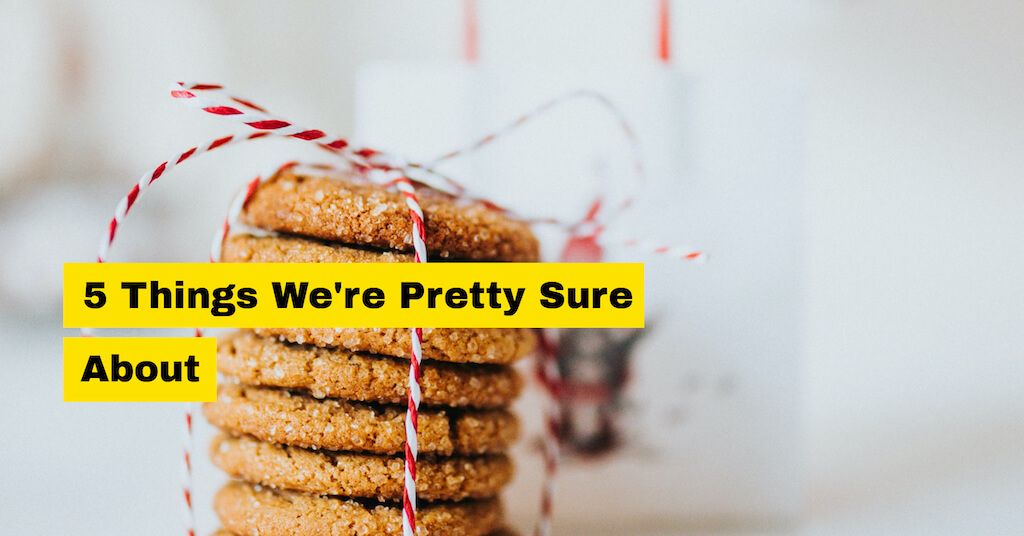
Complexity in this industry goes up when there’s more things we can do with data. Complexity goes down when the demands for data stays the same. The Cambrian explosion in data tools of the late 2000s was in part a response to the sheer scale of data that was suddenly available for analysis. (It wasn’t called ‘Big Data’ for no reason.) It took us around five years before we began paring down some of the complexities of that era.
Would the future mean a simpler data stack for all of us? It’s tempting to answer ‘yes’ — after all, the rise of modern data warehouses mean that we have less things to maintain in our infrastructure, especially when compared to the cube-and-warehouse setups of the past. But the real answer is that it depends. Complexity rises and falls depending on the demands businesses place on data. If the shape of the demand stays the same, there is usually a tendency for vendors to consolidate. To simplify. To make things easier.
One area of rapid change seems to be the infra required to do large-scale machine learning. We may well see a bifurcation in tools: with machine learning-oriented data infrastructure growing in complexity, while business intelligence-oriented tools consolidating — at least over the next few years.
The data modeling approach for the cloud era hasn’t been written yet. Kimball’s four-step data modeling process is too much work to do up front. The Agile Data Warehouse Design was written one year before Redshift launched. Contemporary data teams have moved from ETL to ELT; they have tools that are an order magnitude more powerful than the tools Kimball and Ross had, back when they wrote their book.
We’re still waiting for a practitioner to synthesise a practical, beautiful update to these old ideas. I hope it happens soon.
The data tools change, but the people problems stay the same. When we talk to experienced data leaders, we’re always surprised by how similarly their worldviews present: the job of the data department is to solve business problems. Ideally, to reduce business costs. You want to make sure your analysts understand the business. And you want your business stakeholders to know who to go to for their data questions.
The tools you use may change, but the people problems stay the same.
All things being equal, it’s better to work at a profit center than to work at a cost center. Profit centers are the parts of the business that make money. Cost centers are the parts of the business that exist to help the profit centers make money. Traditionally, the data department has always been seen as a cost center.
This reality is beginning to change — at least for some teams in some companies today. Data teams that ship consumer-facing features like recommendation systems or machine learning models are more often regarded as profit centers. The divide between data teams that are profit centers and data teams that are cost centers will only grow clearer as time passes.
The future’s already here, just not evenly distributed. Snowflake was one of the darling IPOs of the year. Their product raised the stakes for data warehouses everywhere. But the ideas underpinning their technology were hashed out in academia in the late 80s.
It’s tempting to think that ideas emerge, fully formed, from the heads of founders and software engineers. But usually the idea is in the air for a few years before the canonical implementation emerges. Even today, there are small companies experimenting with 'crazy' ideas. One table for your entire data model? That’s being done. Notebooks instead of dashboards? Ditto.
Tomorrow’s hot new tool is likely already here. But if they exist, they probably look like Snowflake circa 2014 — tiny, scrappy, and easy to ignore.
What's happening in the BI world?
Join 30k+ people to get insights from BI practitioners around the globe. In your inbox. Every week. Learn more
No spam, ever. We respect your email privacy. Unsubscribe anytime.