Embrace The Data Schlep First
Every cool data project has some schlep up front. Here's why it's a good idea to keep that in mind.
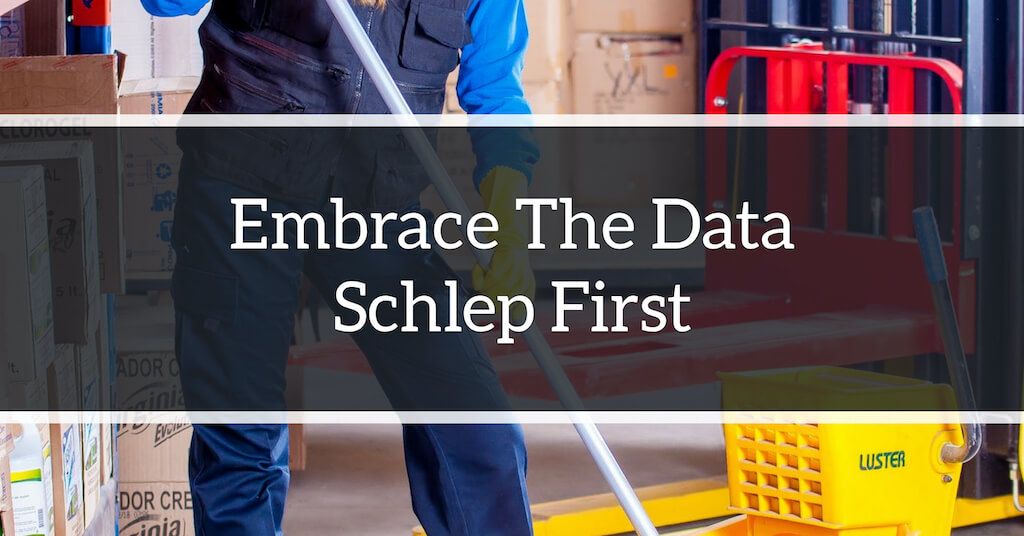
Here’s an uncomfortable truth (or an annoying reminder, if you’re an old hand at this): before you can get anything interesting done in data, you need to get past a period of data schlep first.
What is data schlep? Schlep is an informal term that means tedious, boring work. You can already see where we’re going with this: data schlep refers to all the boring data cleaning and plumbing and transforming that you do at the start of any data project.
At Holistics, we sometimes talk to business leaders in South East Asia who are at the beginning of their digital transformation journeys. More often than not, they talk about exciting data projects that they want to see in their companies — like recommendation systems or predictive modeling, or all the cool applications of machine learning that we read about in the popular press.
And, just about as often, we find ourselves warning them about the data schlep problem that they’ll inevitably face.
“Do you have a data warehouse?” we’d say.
“No,” they’d answer.
“Do you have reporting set up?” we’d ask.
“Not yet,” they’d reply.
“Oh no”, we'd think, and then we would warn them that they’d have to go through a painful period of integrating all the data they have across their organization, collecting things into one place; setting up ELT and warehousing and hiring a team to look after the pipes, before they could do any of the exciting things they were talking about.
We’d like to think that the tool we make helps them with this initial setup period … but then we’re also honest: schlep is schlep. Schlep always exists. It isn’t very sexy, and it always takes time.
Once you understand that schlep exists in every data project, however, you begin to look out for it everywhere cool data projects exist. Here are two stories that demonstrate exactly this.
School Reopenings in COVID-19
Our first story is about a recent Planet Money podcast episode, on economics professor Emily Oster. Oster is a mother of two, and at the beginning of the COVID-19 pandemic, she started a newsletter about making data-driven parenting decisions in the face of extreme uncertainty.
This particular podcast episode focused on a question that Oster tackled over the past few months. She wondered: “Should my kids go back to in-person schooling? Or should they continue to stay at home and do distance learning? What does the data say?”
And just like that, Oster had to tackle some data schlep.
GOLDSTEIN: Here's the thing Emily Oster and lots of other people have been trying to figure out for months: are the places kids congregate — day cares, camps, elementary schools, high schools — are they likely to become super-spreader hubs for COVID? In other words, she wanted to evaluate the risk. But in order to do that, she needed data, and good data on this question were weirdly hard to come by. So finally, sort of out of desperation, a few months ago, Emily decided she was going to try and collect data by herself.
On July 18, she published a newsletter with the subhead Help Get Data!, in which she linked to two simple Google forms to fill out. One was for schools, day care centers, and camps, and the other one was for local governments. Oster describes what happened next:
OSTER: Right. And then like a week later, I had, like, you know, a thousand child care centers. You know, like, it's not like that number is infinity. On the other hand, I'm just a lady with a newsletter, you know? I'm not like — I have no official capacity. And then, you know, I did this, and people were like, OK, like, this is the best — people would be, like, posting, like, this is the best data we have on this. I was like, oh, my God. That's horrible. Like, that's a horrible, embarrassing disaster.
(She means that it’s ridiculous that the best data anyone had on virus spread in child care centers was from a ‘lady with a newsletter’, as she described herself).
A few months after that, when schools started to reopen, Emily realized once again that nobody had good data on schools. Planet Money reported:
Schools might report that they'd had five cases or ten cases, but sometimes they wouldn't say if the kids were remote or in-person. A lot of the time they didn't report how many kids total were at school. They didn't give you the denominator. So there was no way to learn what fraction of kids were infected.
Now, public schools are too big and too complicated for Emily to track on just her little Google Docs. So in August, she gets in touch with the National Association of Superintendents and with a tech company that does data analytics stuff, and they decide they're going to do a bigger, more complex version of the things she had tried with her Google form and newsletter. And schools were interested. Within a few weeks, Emily and her colleagues were getting numbers from schools with hundreds of thousands of students.
When I heard that line — ‘… a tech company that does data analytics stuff’ — I sat up in my seat and smiled. Oster had found someone — Qualtrics, as it turned out — to outsource the data schlep to! Yay!
The result of this adventure was quite consequential. With Qualtrics’s help, Oster had a dashboard set up that tracked COVID infections in schools. She discovered that schools were not super-spreader locations — they were not vectors that could spread infection into the broader community. Rather, the data seemed to say that kids were getting infected outside and bringing it into the schools. In her newsletter on the results, she wrote:
In students, we see a rate of between 0.078% (that’s 0.78 cases in 1000) for confirmed, 0.23% (2.3 cases in 1000) for confirmed plus suspected. For staff, these numbers are 0.15% and 0.49%.
Planet Money concluded:
Emily says this is a good sign. It suggests that schools, and especially elementary schools, are not petri dishes where everybody is getting sick and spreading the disease, you know, out into the community. Still, the data are very preliminary. It's a relatively small sample of schools. It's only schools that have volunteered to do it. And it's only been up and running for a month or so. But Emily says data are finally starting to come in from other places. Texas is doing a lot of reporting on its own now, and its reporting not just cases, but total number of students. And it shows similar rates to what Emily's dashboard shows.
Oster looked at this data, and then began to dig into the academic literature on the efficacy of distance learning for kids. She discovered that the results weren’t great. Distance learning wasn’t working very well. She also learnt that superintendents across the country were reporting high no-show rates for remote classes. She could see her kids falling behind.
And so Oster was faced with a difficult decision: on the one hand, she could wait out the entire pandemic and not send her kids back to school until COVID blew over. But how long would that take? It could be an entire year or more. On the other hand, Oster knew that her decision to keep her kids at home came with a cost — they would fall behind.
So what did she do? Emily Oster framed the question, thought about how to mitigate the risks, evaluated the drawbacks based on her data, weighed that against the benefits, and then finally … decided to send her kids back to school. And she wrote an article in The Atlantic, titled Schools Aren’t Super-Spreaders.
With her data collection work, she’s ignited a debate that’s still going on in the US today. And she did it by getting past the schlep.
Data Schlep in Improving Surgical Outcomes
Our second story is from 2014. It’s a piece by James Somers, titled Should Surgeons Keep Score?
The article took an in-depth look at an attempt to improve surgeon performance. Andrew Vickers, a biostatistician at the Memorial Sloan Kettering Cancer Center, started looking into the question of surgeon performance in the late 2000s, eventually starting a software project called Amplio in 2009. The goal of the project? Tell surgeons just how well they were doing.
Vickers likes to put it this way. His brother-in-law is a bond salesman, and you can ask him, How’d you do last week?, and he’ll tell you not just his own numbers, but the numbers for his whole group.
Why should it be any different when lives are in the balance?
Somers continues:
The first big task with Amplio, he (Vickers) said, was to get the data. In order for surgeons to improve, they have to know how well they’re doing. In order to know how well they’re doing, they have to know how well their patients are doing. And this turns out to be trickier than you’d think. You need an apparatus that not only keeps meticulous records, but keeps them consistently, and throughout the entire life cycle of the patient.
That is, you need data on the patient before the operation: How old are they? What medications are they allergic to? Have they been in surgery before? You need data on what happened during the operation: where’d you make your incisions? how much blood was lost? how long did it take?
And finally, you need data on what happened to the patient after the operation — in some cases years after. In many hospitals, followup is sporadic at best. So before the Amplio team did anything fancy, they had to devise a better way to collect data from patients. They had to do stuff like find out whether it was better to give the patient a survey before or after a consultation with their surgeon? And what kinds of questions worked best? And who were they supposed to hand the iPad to when they were done?
Only when all these questions were answered, and a stream of regular data was being saved for every procedure, could Amplio start presenting something for surgeons to use.
And just like that, the first major part of the Amplio project was data collection and consolidation — schlep, if you would.
It took them years.
In 2014, the time when Somers researched and wrote the piece, Amplio was starting to affect real patient outcomes. It gave surgeons access to a private, personalized dashboard that showed them where they stood on a series of plots. A surgeon’s performance would be displayed using a red dot. Blue dots would represent the relative performance of all the other surgeons in their group.
You can slice and dice different things you’re interested in to make different kinds of plots. One plot might show the average amount of blood lost during the operation against the average length of the hospital stay after it. Another plot might show a prostate patient’s recurrence rates against his continence or erectile function.
There’s something powerful about having outcomes graphed so starkly. Vickers says that there was a surgeon who saw that they were so far into the wrong corner of that plot — patients weren’t recovering well, and the cancer was coming back — that they decided to stop doing the procedure. The men spared poor outcomes by this decision will never know that Amplio saved them.
Again: incredibly novel project. Huge impact. Lives saved.
And years of schlep.
Wrapping Up
So what do we take away from this? I think one obvious takeaway is that if you read any cool story about data today, you should look, carefully, for the hidden schlep behind all the sexy data-driven analysis. You'll have to be a bit of a data nerd, but odds are good that you're one — you're reading this blog, after all.
And there are a number of second order implications.
Schlep means that you'll have to think about political cover as you’re working on data projects. Most business stakeholders won’t understand why things take so long. If you’re about to embark on a brand new digital transformation effort, you might want to think of smaller, intermediate goals with lower levels of schlep — so you can deliver business value faster to your people.
Of course, if by some chance you’re a business person reading this, you should probably recognise that any data project comes with some amount of schlep up front — and as a general rule, the more ambitious the project, the higher the levels of schlep.
Finally: feel free to steal the stories here, to put in presentations or to tell your bosses in meetings. You may want to remind them that it may take months before the full scope of their data transformation projects bear fruit. Godspeed, and good luck.
What's happening in the BI world?
Join 30k+ people to get insights from BI practitioners around the globe. In your inbox. Every week. Learn more
No spam, ever. We respect your email privacy. Unsubscribe anytime.