The Six Ways People Deflect Inconvenient Data
We take a look at an exhaustive list of reasons people give when they want to ignore anomalous data.
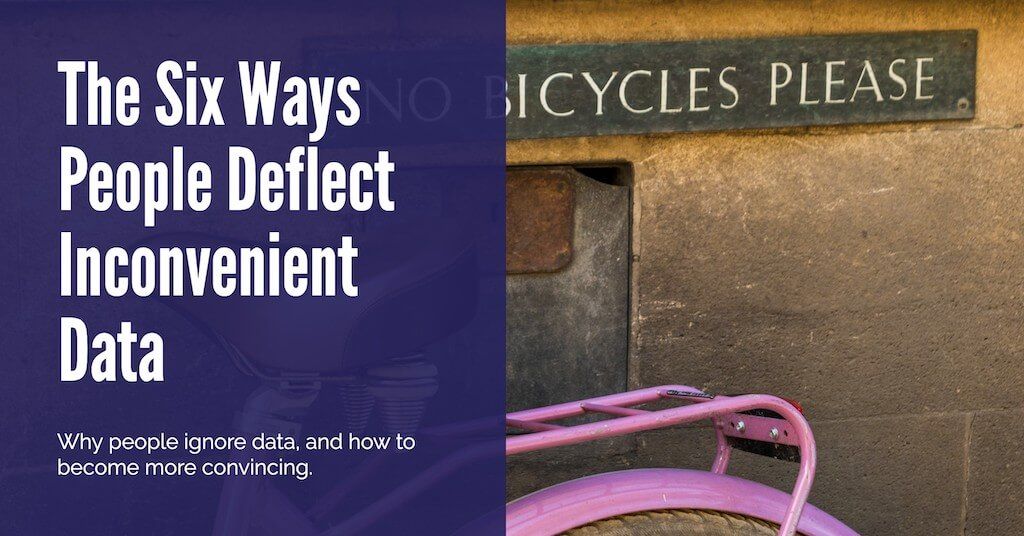
Let’s say that you’ve presented a business leader with some data, and they set it aside. You ask why.
“Oh it’s obviously wrong,” they say, brow furrowed. “It’s the result of an unreliable data source. Remember that incident from back when —“
“That’s two years ago.” you point out. “We spent a whole day fixing it, and we now have a process to make sure that doesn’t happen again.”
“Oh, it has, hasn’t it?” the manager says, wrinkling their nose. “Well, I don’t trust it.” You shrug and leave; the report isn’t particularly important to your job. Later you learn, from Mary in marketing, that the manager didn’t trust the data because it violated a hypothesis he had about a particular marketing campaign that he wanted; he went ahead with it anyway.
A few weeks later you sit in on a planning meeting and notice the manager triumphantly talking up the results of some other campaign. You lean over and grab a copy of one of the printouts, and you realise that it’s from the same dataset you’d presented from — exported from the exact same systems that produced the first report. Only this time the data confirms what the manager wants to believe, and he's conveniently forgotten about the ‘data reliability issues’ … because he wants to crow about it.
This should be familiar to you. In fact, it should be familiar to all of us — what data professional hasn’t seen examples of motivated reasoning?
The more interesting question to ask, though, is “how do people reject inconvenient data?” and “when do they accept data?”
The answers to this question are frankly more interesting than they deserve to be. And they come from a 1993 paper by Clark Chinn and William Brewer of the University of Illinois at Urbana-Champaign.
The Six Ways That People Ignore Data
Chinn and Brewer’s paper is titled The Role of Anomalous Data in Knowledge Acquisition, and it studied the way science students reject anomalous data when learning scientific theories.
However, the results Chinn and Brewer found apply to more than just science students — as part of their argument, the researchers presented examples from the history of science to show that these responses are fundamentally human, and that the way science students react to anomalous data are pretty much the same ways that scientists have reacted to new developments throughout history.
Presumably, these are the same ways that non-scientist adults react to anomalous data today.
Chinn and Brewer also claim one more thing that is useful to us — they claim that the list of possible deflections that they present are exhaustive. I mostly take this assertion to be true. In the two decades since they first published their paper, no other researcher that I know of has come up with additions. (To be fair, I’ve only done a cursory search — but I’ve read multiple papers downstream of this one and they all sort of take it as a fixed set). This means that it’s probably really just six ways that people deflect anomalous data, and that’s good to know.
These are the seven possible responses to anomalous data:
- They may ignore the data. (“I know you think the report is important to look at but I disagree, I don’t want to hear about it.”)
- They may reject the data. (“Bah! This must be the result of shoddy experimental design!”)
- They may find a way to exclude the data from an evaluation of the theory or mental model. (“Oh, this data is interesting, but it isn’t of any concern to us — this is sales’s problem.”)
- They may hold the data in abeyance — meaning they suspend it temporarily from consideration. (“Ahh, this is probably noise, though it’s strangely persistent. Anyway it shouldn’t affect us now. We’ll worry about it later.”)
- They may reinterpret the data while retaining their theory or model. (“Ok I accept that we’re seeing a dip in sales in June, but this is simply part of a yearly pattern. See, sales last year was also down in June!”)
- They may reinterpret the data and make only peripheral changes to the theory or model. (“Fine, maybe our sales is doing worse than normal, even given the yearly June dip, but that’s because our three top salespeople were sick. Things will get better next month, you’ll see.”)
- Finally, they may accept the data and revise the mental model (this is obviously not a deflection; e.g. “Ok, we’re totally screwed. All hands on deck, something is clearly wrong with our sales”).
Chinn and Brewer point out that these seven responses may be reduced to a mere three questions:
- Does the person accept the data as valid? If they reject the data, there’s no need to generate an explanation for it.
- Can the person provide an explanation for why the data is accepted or not accepted? If they want to reject it and preserve their mental model, they have to come up with a plausible argument for why it doesn’t apply. (Conversely, if they want to believe in the data, they have to come up with a plausible reason for why it does).
- Does the person change his or her prior theory? A person can accept the data as valid and generate an explanation for the data, but may opt to stick to the prior theory, or at most make peripheral changes. This is the question that we’re most interested in, of course.
And then they go on to say that the three questions aren’t presented in a particular order — they may be answered in parallel for all we know. The point is simply that anyone who generates one of the seven responses must also generate an answer to all three questions.
For what it’s worth, I find the three questions easier to remember.
What Factors Affect Belief?
What, then, makes a person more willing to change their mind when presented with anomalous data? I mean, apart from motivated reasoning, like our manager in the opening of this piece, people can and do reject anomalous data for all sorts of valid reasons.
Chinn and Brewer give us four factors to think about:
- The characteristics of prior knowledge. This includes a) how entrenched the prior model is (the more actions or ideas are dependent on the model, the more entrenched that model becomes), b) if the prior model is of an ontological nature (e.g. is so deeply held that it is almost built into how they see the world — like ‘marketing can’t possibly do anything useful, and sales does all the work’), c) if the person has epistemological commitments (e.g. they have certain hard-held rules about what they consider true and false — for instance, ‘qualitative data is anecdotal evidence, and anecdotal evidence doesn’t count!’), and d) if they happen to possess background knowledge that may justify or discredit the data (e.g. things they know that they bring to bear on their judgment of the data — “I have it on good authority that if you type Google into Google, you can break the internet …”)
- The characteristics of the new theory (or explanation). This includes a) the availability of a plausible alternative explanation for the data, and b) the quality of that alternative explanation. Naturally, anomalous data with no alternative theory attached doesn’t get as much play as anomalous data with an alternative theory; the best possibility is anomalous data with a compelling alternative explanation.
- The characteristics of the anomalous data — Is it a) credible? If it’s not credible, they can reject it. b) Is it ambiguous? If it’s ambiguous, they can usually reinterpret the anomalous data to fit into their existing mental models. And, c) are there multiple pieces of anomalous data? The more pieces of anomalous data you have, the more difficult it becomes for them to brush it aside.
- Finally, the individual should engage in sufficiently rigorous processing strategies — by which the authors mostly mean ‘do they take the time to properly walk through the evidence?’ Which is great if you’re reading a report and have the time to mull it over, but less great if your boss is yelling at you and you’re hungover from drinks the night before.
At the very end of the paper, Chinn and Brewer say that these factors are useful to science educators because you may invert them when teaching new scientific theories to kids.
I think this applies to data folk as well — the next time you’re facing someone who is reluctant to embrace what the data tells them, break down why they believe what they believe by looking at the three questions above, and then work through the checklist of four factors in order to figure out what else you need to give them to believe you.
Of course, this is an ideal situation, where hopefully you're faced with a reasonable manager — someone who wants to get to the bottom of things. If you're up against a business leader who's determined to stick to motivated reasoning — then, well, good luck.
What's happening in the BI world?
Join 30k+ people to get insights from BI practitioners around the globe. In your inbox. Every week. Learn more
No spam, ever. We respect your email privacy. Unsubscribe anytime.