How Amazon Uses Input Metrics
How Amazon uses input metrics, a data pipeline is a materialized view, and the case against self-service data and analytics.
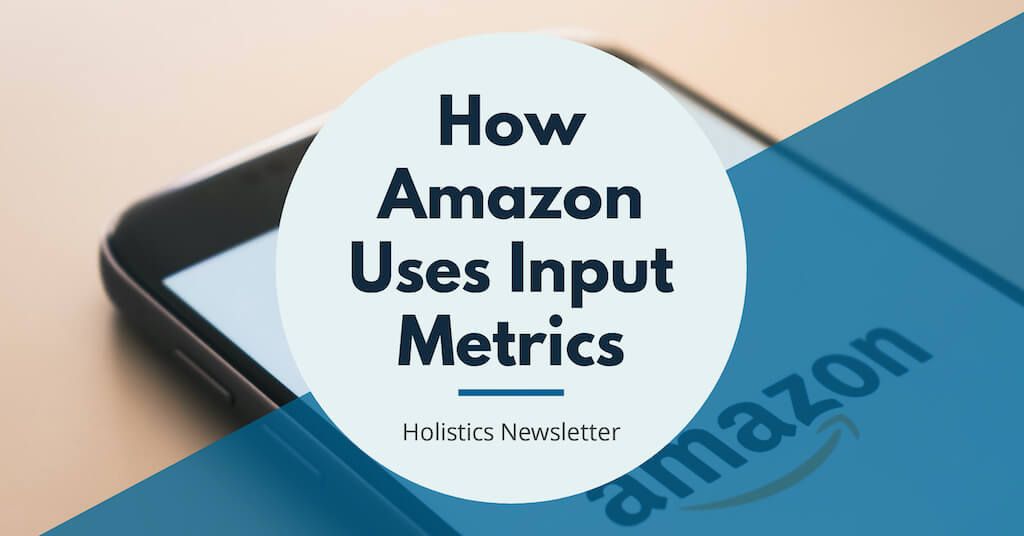
This newsletter was originally sent out on the 24th of February 2021. Sign up here if you'd like more like this.
Inner Join is Holistics's weekly business intelligence newsletter. This week: why Amazon uses input metrics, a data pipeline is a materialized view, and the case against self-service data and analytics.
Insights From Holistics
How Amazon Uses Input Metrics
I mentioned last week that I'm working my way through Working Backwards, a book by Colin Bryar and Bill Carr about how Amazon really works. If you're in business intelligence, the good bits about data and analytics and metrics are all in Chapter 6.
It's amazing. Go read it.
We're going to summarize the entire chapter on our blog in the near future, but I wanted to excerpt a small bit here:
Jeff and I (Colin) once visited a Fortune 500 company to meet privately with the CEO in his office. During our meeting, an assistant dashed in and handed the boss a sheet of paper. The CEO glanced at it, waved it at us, and proudly said, “Our stock is up 30 cents this morning!” His mood brightened, as if he had personally caused the rise.
As we drove to our next meeting, Jeff said, “There’s nothing that CEO did to cause that 30-cent blip in the stock price.” I agreed, and added that I wouldn’t be surprised if the assistant had thrown multiple printouts in the recycle bin that morning when the blip wasn’t so big. Would the same scene have played out if the share price had dropped 30 cents? The deeper lesson, one that we’ll explore in this chapter, is this: share price is what Amazon calls an “output metric.” The CEO, and companies in general, have very little ability to directly control output metrics. What’s really important is to focus on the “controllable input metrics,” the activities you directly control, which ultimately affect output metrics such as share price.
The way that Amazon does this is pretty intense. It happens every week, at something called The Weekly Business Review (WBR), where all the valuable input and output metrics (along with a few exception reports and customer anecdotes) are presented to company leadership in a large deck.
The authors of Working Backwards aren't kidding when they say that all the valuable metrics are presented — the deck is organized with the exact same metrics as the previous week, in the exact same format, using the exact same fonts, with the exact same symbols, in the exact same order, so that the leadership can walk through the numbers from the perspective of a typical customer from site visit to product ordering to fulfilment to delivery.
But the big thing I want to highlight here is Amazon's clear delineation between input and output metrics.
The authors make a case for why 'controllable input metrics' are so important — when you're looking at one, you know exactly what you need to improve. This is in contrast to, say, output metrics like revenue and stock performance and NPS scores — if you discover that these numbers are trending downwards, well, what can you do to improve them? It's impossible to say. So, while Amazon's business leaders are familiar with output metrics, it's the input metrics that they are expected to pay attention to.
What I loved about Working Backwards is the description of the process that Amazon uses to get to good input metrics. I'll excerpt an illustrative bit:
One mistake we made at Amazon as we started expanding from books into other categories was choosing input metrics focused around selection, that is, how many items Amazon offered for sale. Each item is described on a “detail page” that includes a description of the item, images, customer reviews, availability (e.g., ships in 24 hours), price, and the “buy” box or button. One of the metrics we initially chose for selection was the number of new detail pages created, on the assumption that more pages meant better selection.
Once we identified this metric, it had an immediate effect on the actions of the retail teams. They became excessively focused on adding new detail pages—each team added tens, hundreds, even thousands of items to their categories that had not previously been available on Amazon.
(...) We soon saw that an increase in the number of detail pages, while seeming to improve selection, did not produce a rise in sales, the output metric. Analysis showed that the teams, while chasing an increase in the number of items, had sometimes purchased products that were not in high demand.
Oops.
So far, this seems like a typical Goodhart's Law story — you define a metric and then you realise that your company is optimizing for the wrong thing.
But what's instructive is Amazon's use of the dual nature of the input and the output metric to correct for this:
When we realized that the teams had chosen the wrong input metric—which was revealed via the WBR process—we changed the metric to reflect consumer demand instead. Over multiple WBR meetings, we asked ourselves, “If we work to change this selection metric, as currently defined, will it result in the desired output?” As we gathered more data and observed the business, this particular selection metric evolved over time from
- number of detail pages, which we refined to
- number of detail page views (you don't get credit for a new detail page if customers don't view it), which then became
- the percentage of detail page views where the products were in stock (you don't get credit if you add items but can't keep them in stock), which was ultimately finalized as
- the percentage of detail page views where the products were in stock and immediately ready for two-day shipping, which ended up being called 'Fast Track In Stock'.
Bryar and Carr are quick to say that this process is repeated across all metrics in Amazon — you keep in mind an output metric, and then you evolve your input metrics, through trial and error, until you get to the right set of correct, controllable inputs.
In essence, this process of test and debate is how Amazon gets around Goodhart's Law!
I thought that was a cool idea indeed.
Insights From Elsewhere
Why I've tracked every single piece of clothing I've worn for three years — I've been sitting on this funny-but-useful piece for a few weeks now. Olaf Hoverfält did a ridiculously deep dive into what his clothes truly cost, and learnt a ton about performance, sustainability, and taste in the process. His chosen metric? 'Cost per wear' — which is the purchase price divided by the cumulative number of wears for that single piece of clothing!
The Case Against Self-Service Data and Analytics — Kat Hempstalk says that she used to believe in self-service data and analytics, but now she thinks twice about recommending this approach. The argument she makes is fair, and nuanced — she points out, rightly, that there are very good business reasons to keep the data behind a fortress.
I have one other point to add to her argument — in Working Backwards, the authors explain that in Amazon, ultimate responsibility for truthful and accurate metrics lies with the finance team(!). That team is empowered by Bezos to go after the truth and only the truth, and this structure exists so that individual business units are not able to fudge their numbers.
The principle matters more than the actual implementation, of course. Hempstalk's argument hews to similar lines. Sometimes there are benefits to limited access.
A Data Pipeline is a Materialized View — Nicholas Chammas takes the core transformation paradigm in an ELT system and explains it from first principles: yes, a data pipeline in today's data world is essentially just a materialized view.
This post should give you a good understanding for the atomic concepts behind the approach — an approach that also happens to underpin Holistics's modeling layer.
That's it for this week! If you enjoyed this newsletter, I'd be very appreciative if you forwarded it to a friend. And if you have any feedback for me, hit the reply button and shoot me an email — I'm always happy to hear from readers.
As always, I wish you a good week ahead,
Warmly,
Huy,
Co-founder, Holistics.
What's happening in the BI world?
Join 30k+ people to get insights from BI practitioners around the globe. In your inbox. Every week. Learn more
No spam, ever. We respect your email privacy. Unsubscribe anytime.