Data as a Thermostat, the Necessity of Reorganizations, and Data Generalists vs. Data Specialists at Wayfair: An Interview with Nachiket Mehta
Designing Data Organizations is brought to you by Holistics, where we delve into the intricate process of creating and optimizing data teams within organizations.
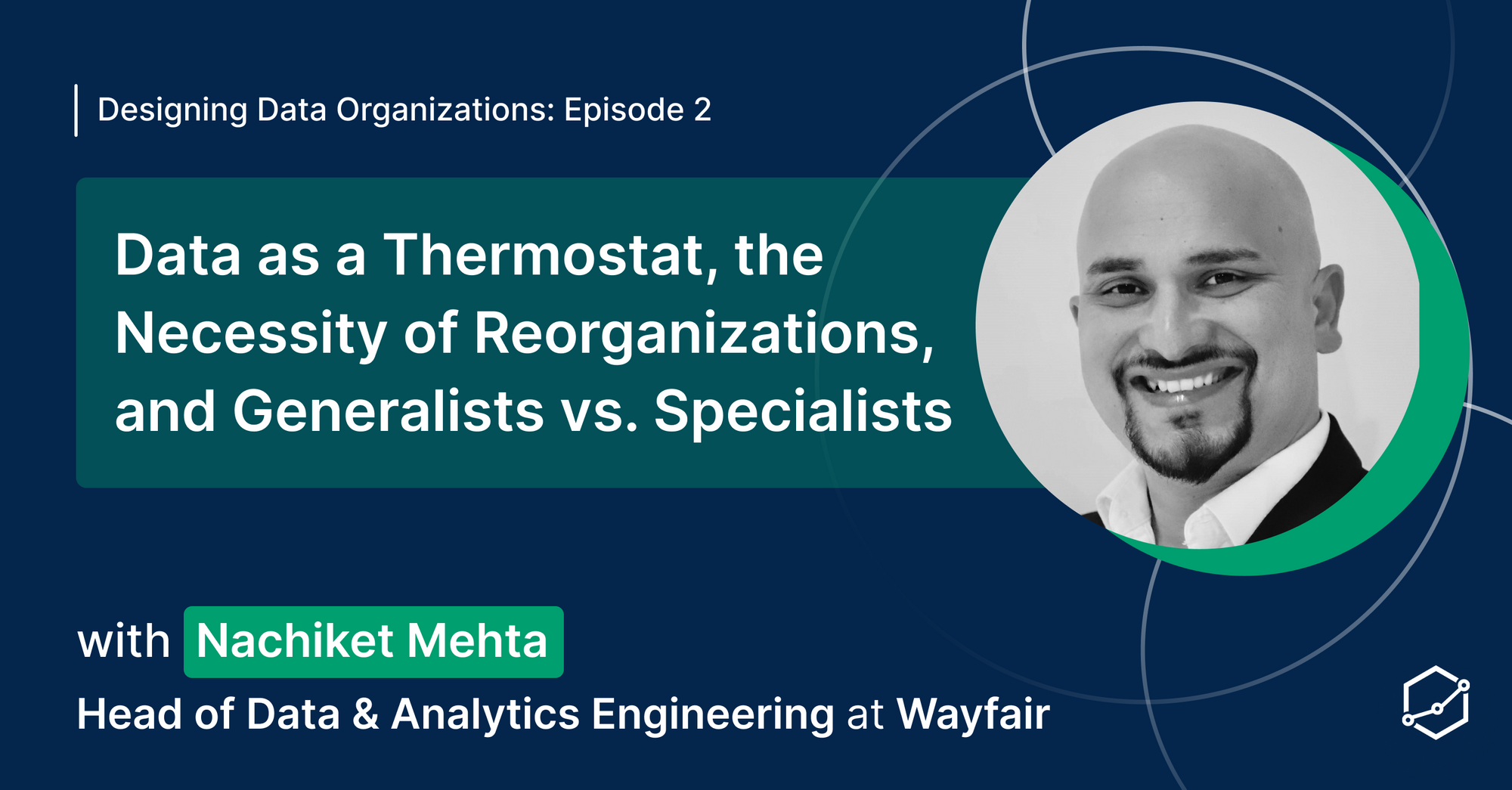
For this week’s episode of Designing Data Organizations, we spoke with Nachiket Mehta, Head of Data and Analytics Engineering for Global Operations at Wayfair, who has been with the organization since 2019.
Designing Data Organizations is brought to you by Holistics, where we delve into the intricate process of creating and optimizing data teams within organizations. In this article series, we sit down with seasoned data leaders from various industries to uncover their strategies, challenges, and success stories in designing effective data teams.
Founded in Boston, Massachusetts in 2002, Wayfair is a leading e-commerce company specializing in furniture and home goods. It offers an extensive selection of over 40 million items across home furnishings, décor, home improvement, and more. As of 2024, it serves over 22 million active customers per year. Wayfair’s commitment to transforming the way people shop for their homes has positioned it as a key player in the online retail space.
• At Wayfair, data organizations are federated primarily under technology domains, and sporadically under business domains.
• Data roles are split into the “data producing” side (e.g. frontend application engineers), the “data consuming” side (e.g. data analysts), and data engineers who build and develop data products and platforms in between them. However, they are implementing a decentralization strategy.
• Among Wayfair’s 10,000+ corporate employees, almost half of them use data everyday.
• Certain talents, such as ontologists, data platforms engineers, and data product managers, are niche and difficult to find.
• At large enterprises, data organizations need to be scalable.
• Wayfair follows a Single-Threaded Ownership (STO) approach for setting and communicating biannual strategic priorities.
• Reorganizations are occasionally necessary to maximize business impact.
• Communication is key to managing the impact of reorganizing.
• AI is driving an unprecedented speed of change within the analytics industry.
• Mehta anticipates that data responsibilities within companies will become more and more distributed.
Data as a thermostat
Gabriel Zhang: Nachiket, thank you for joining us! For starters, can you give me a snapshot of the data organization that you’re leading at Wayfair?
Nachiket Mehta: At a large company like Wayfair, we don’t have a central data office. Instead, we have multiple data organizations that are federated under a domain, and all of them ultimately report to the CTO.
I personally own one of the largest organizations for data and analytics within the Operations domain, which covers areas such as end-to-end supply chain management, customer experience, data platforms, and so on.
We have several data engineers who work on traditional tasks, such as maintaining historical data pipelines to bring data into the data warehouse, and software engineers who build the platforms and applications. However, our organization works on a lot of data problems beyond this traditional framework.
Can you give me an example of a unique data problem that your organization works on?
To simplify things a bit, Wayfair has data-producing teams, and data-consuming teams. Data producers are the engineering teams who build Wayfair’s frontend applications such as e-commerce Storefront, Supplier platforms, Supply Chain applications, etc.
One of the key questions faced by my organization is, “How can we empower these application engineering teams, so that they not only own their applications as products for their stakeholders, but also their data as a product?” To do that, there needs to be clear ownership of data, the quality of the data needs to be guaranteed, and so on.
To solve these issues, we have embedded data engineers with the data producers to promote ‘data as a product’ mindset with federated governance and best practices. The ownership of the data remains with the SMEs of the domain and is not delegated downstream to a centralized data team to fix the source data quality issues.
The data platforms team builds various tools to expedite data movement velocity from their origin to the destination. They are also responsible for building and maintaining certain data governance tools, such as data contract management, data catalog, and data observability, to provide a data marketplace experience to the users.
Critical to a lot of these automated processes is an Ontology and Knowledge Graph, which is led by a small group of ontologists within the organization.
“One of our core principles is that data is our thermostat.”
The teams that you’ve described so far, both within and outside your immediate organization, sound like the so-called “data producing” teams.
Tell me more about the data teams who work on the “data consuming” side.
The “data consumer” facing teams in my organization are well-versed in the data engineering and business domain expertise. They stitch together the ‘high-precision data’ from multiple domains into an ontological object model so that the data consumers get consistent access to this data to support various analytical use cases, such as executive reporting, end-to-end visibility applications, and advanced analytics with AI/ML algorithms.
Like most companies, Wayfair has data analysts and business analysts. They’re a diverse group with expertise across many horizontal business domains.
There are also data scientists and ML (machine learning) engineers. Data scientists are typically the PhDs who build science models, but in practice, they deal with a lot of foundational data problems too. Another thing that my organization does is to help these data scientists resolve issues of data quality, so that they can focus on model development and other operational responsibilities.
Last but not least, there’re the data ops and ML ops teams. And in a nutshell, that’s what the landscape looks like today.
Wayfair is a large corporation. I imagine that its data ecosystem looks very different from that of smaller companies. How many people are involved in this ecosystem?
Out of Wayfair’s 17,000+ employees and contractors, about 3,000 consist of technical staff. Within this group, the total number of data engineers, BI engineers, data scientists, ML engineers, etc. is, I think, 200 maximum.
However, one of our core principles is that data is our thermostat. That means that, regardless of which domain you sit in, data is critical to measuring and guiding your team’s project or business performance. As a result, there’s a very large group of folks outside of this core group of data contributors who nonetheless use data indirectly as they drive business decisions.
We have about 8,000 people working in Operations - folks who manage end-to-end supply chain, customer service, supplier support, and so on. Around 2,500 people in Commercial org assist catalog management, B2B business and global sales, category management, marketing, etc. The other business functions, such as talent/HR, finance, legal, etc. are embedded under the Enterprise org.
Within all of the departments that I just mentioned - Operations, Commercial, and Enterprise - they have their own business analysts and data analysts who write build reports, create data visualizations, and write ad-hoc queries that respective tech organizations, like mine, have built.
At least within my core domain (Operations), around 30% of all Operations staff access our data products directly or indirectly. Other domains, outside my core domain, also use our data to understand things like customer order visibility, supplier visibility, fraud detection, and customer NPS scores.
Data Generalists vs. Specialists
Thank you for the thorough sketch of the data landscape at Wayfair! Let’s return to your organization again.
What’s the degree of specialization in your organization? Would you say that it consists mainly of specialists or generalists, or some mix of both?
I think it’s a mix of both; we have a good balance between specialists and generalists. Our specialists consist of data scientists, data engineers, data infrastructure specialists who work on some pretty complex problems. Their talents are very difficult to find.
And then we have generalists - your BI analysts and other business-facing consultants - who have much broader skill sets that allow them to support multiple departments, and tackle business problems on the fly.
“It’s really hard to find people who understand how data can be monetized, or how to build a data marketplace.”
You just commented that there’re specialists with skills that are very difficult to find. Would you share examples of specific roles in your organization that you’ve found challenging to fill or replace?
One that comes immediately to my mind are ontologists. These are typically individuals with degrees in information science, library science, etc. They are capable of building library management systems and knowledge graphs. It’s a very niche and rare talent on the market, and if you find them, you must retain them as best as you can.
On the data engineering front, I’d say data platforms engineers. I always say that there are two types of data engineers. In the first category are people who come from traditional business intelligence roles, and who excel at data analysis. Typically, they got into developing ETL (extract, transform, load) pipelines, and then converted into data engineers.
In the second category are people who come from a core software engineering background, and who have deep knowledge in things like Java programming. They have very specialized skills required for data platforms where scale and performance really matter, such as the constant streaming of real-time data.
Last but not least, good data product managers are also really hard to come by. The idea of treating data as a product is still a relatively new concept; most PMs merely treat it as a byproduct. A good data product manager understands how data can be monetized, or how to build a data marketplace.
Scaling Data Organizations
You’ve led many different organizations, both before and at Wayfair. Have you found any data organizational structures that have worked particularly well?
A lot of this depends on the needs of the business. I’ve tried different organizational structures over time. Historically, I had data organizations that closely mirrored our data consumers; so if they were organized in a certain way, we’d do the same. As a result, there’d be a one-to-one connection between the senior leaders, the managers, and the individual contributors.
But this isn’t a scalable approach; when the number of consumers is in the thousands, you inevitably have a one-to-many ratio between data support and consumers.
About four years ago, we structured the data organizations at Wayfair so that they would correspond to domains or subdomains, meaning internal business units such as Large Parcel, Small Parcel, Wayfair Delivery Network, Last Mile, and so on. Within each domain, we’d serve dozens of atomic teams.
Later, as the company grew, this structure was abstracted even further, whereby all business activities that occur before a customer places an order fall under “inbound supply chain”, and everything that happens after falls under “outbound supply chain”, and our data organizations were iterated again to reflect that.
“...the planning process for any internal tech transformation at Wayfair begins at the C-level…”
What does the line of communication look like between your organization and your stakeholders? How do you align on business objectives?
Firstly, the planning process for any internal tech transformation at Wayfair begins at the CTO level, focusing on a list of overarching objectives we call Mega Rocks and Big Rocks. Right now, a lot of our initiatives are aimed at evolving our tech architecture from big monolith to decoupled.
In terms of execution, we apply a Single-Threaded Ownership (STO) approach. Every six months, business and engineering leadership meet to review and set priorities for the next two quarters.
During these meetings, the leaders also figure out their cross-functional dependencies. For example, if one organization is solving a problem that requires data, then it will identify its specific dependencies on data organizations.
Next, we have monthly business reviews (MBRs) and weekly business reviews (WBRs) to set alignments between organizations and articulate how objectives are broken down into projects.
Last but not least, we send weekly flash reports that communicate the status of each initiative to the STOs that we belong to.
The necessity of reorganization
Wayfair is a large corporation. I imagine that its data ecosystem looks very different from that of smaller companies. How many people are involved in this ecosystem?
In most situations, they’re ultimately driven by business needs. Unfortunately, what’s happened sometimes is that leaders in the past created justifications just so they can get a budget approved, or create bigger teams. But then you come to realize that you don’t need a big team to create a big impact.
Most of the data and analytics teams fall within this category, in the sense that it’s possible for them to solve big problems at scale with a small but very specialized team, supported by an auxiliary team of generalists.
There are three possible levers you can move when you need to shake things up: technology, process, and people. Changes in technology are the fastest to implement, while processes require more time. But changing people and their culture, mindset, and problem-solving approaches… takes a long time.
If you have well-organized teams, who have good camaraderie and working relationships, you don’t want to disturb that unless there’s genuine cost pressure.
So I’m aware that you’ve led teams through reorganizations in the past. I wonder if you’d be willing to share a few anecdotes.
Sure, I’ll give you an example of change that’s happened gradually. We supported a lot of consumer-facing teams that are unique to Wayfair: these are the units like Small Parcel, Large Parcel, Wayfair Delivery Network, Last Mile, etc. that I mentioned earlier.
Over the last few years, we began to center our attention on the quality of data production for these teams - what we termed a “shift left approach” - because that’s the root of a lot of data problems.
“Changes in technology are the fastest to implement, while processes require more time.
But changing people and their culture, mindset, problem-solving approaches… that takes a long time.”
We partnered with another data organization - GAT (Global Analytics Tech) - who took over the downstream side of things, while we moved even more into the platform side of things. We then built a curated data layer which, in turn, powered business intelligence and data science teams.
To ensure the right balance of skill sets for this initiative, I’ve converted and restructured a team that includes pure software engineers - front-end and full-stack developers with essential Java programming skills.
We also have a product manager who works with our internal stakeholders to identify their pain points, and how we can increase the velocity of data ingestion into the curated data layer.
You mentioned that when there are good working relationships within a team, you’d want to maintain that. But obviously reorganizations happen all the time, due to factors like the ones you just mentioned.
And there’s bound to be some negative impact, whether that’s a sense of uncertainty, or people’s career trajectories getting disrupted. How do you, as a data leader, massage the frictions that come with reorganizations?
So, communication is going to be key here. First, you have to accept that the priorities are going to change. When communicating to external stakeholders, you’ll have to set expectations and get their buy-in. Internally, you’ll have to revise the team charters and set clear boundaries on newly defined roles and responsibilities.
Nevertheless, moving forward, it’s important to give them the freedom to innovate. You can articulate the mission, call out your core tenets and values and things like that, but it’s important to promote a culture of having solutions come from within the team, instead of imposing them top-down.
AI Becomes Mainstream
Let’s turn towards the future. I can personally say that the analytics industry in 2024 looks very different since I first started working almost a decade ago.
For example, analytics engineers didn’t really exist until about five years ago. Now, thanks to tools like dbt, it’s a completely new function in itself, along with data engineers and data analysts. What else do you think will change?
That’s a good question; every data leader wishes they have a crystal ball. Historically, the data space used to consist of traditional data warehouses, which were supported by a business intelligence organization. Then the cloud computing era arrived - thanks to Databricks, Snowflake, and others - and everyone moved into the cloud.
Then there’s the concept of bundled versus unbundled services. Previously, giants like Informatica, SAP, and Oracle provided most data services, but now people are picking and combining services for very specific products. You have the so-called “modern data stack”, like dbt, Looker, Metabase, Airflow, and whatnot.
“Where there is pain, there’s an opportunity, and people who will create solutions based on those pains.”
We have been using AI at Wayfair for the last decade; we use a lot of models for things like fraud detection, but the growth of AI is going to accelerate.
One emerging area will be the determination of data authenticity, or the lineage of products. Like, which video or image is authentic? Which song is original, and which one is generated by LLMs (large language models)?
In another two years, workflows will look a lot different depending on how generative AI evolves. We used to write hundreds of lines of code to define data pipelines, right? Now there are no-code platforms that do that for you. Where there is pain, there’s an opportunity, and people who will create solutions based on those pains.
Yeah, the speed at which AI is evolving is mind-boggling. Surely, it’s going to alter data organizations drastically.
Do you think data functions will get smaller? Do you think some roles will disappear, or get consolidated and become the same thing?
Yeah, there’s definitely going to be an impact. And again, it depends on the maturity of the organization. I know many organizations that are moving quickly and jumping into the generative AI craze for the sake of saying, “We are doing this!”.
However some of these organizations don’t have sufficient data maturity, and they’ll need to invest in their data foundations and excel at basic best practices first. Nevertheless, they’ll move much closer to the platform approach, i.e. selecting the tools that solve specific problems instead of just building a lot of infrastructure.
However, for the organizations that have invested into the right resources over the last few years and built a good foundation, they’ll reap greater benefits from enablement.
Regardless, my prediction is that data or analytics won’t be a strongly distinct function, it’s going to be everyone’s responsibility. I talked about Wayfair’s landscape earlier, where 40 to 50% of business users use data indirectly. That’s going to be the norm, and more and more organizations are going to be restructured to reflect that.
Thank you so much, Nachiket. This interview has been way more comprehensive than I expected!
What's happening in the BI world?
Join 30k+ people to get insights from BI practitioners around the globe. In your inbox. Every week. Learn more
No spam, ever. We respect your email privacy. Unsubscribe anytime.